Projects and opinions from the world of Citizen Science. Because getting started is easy.
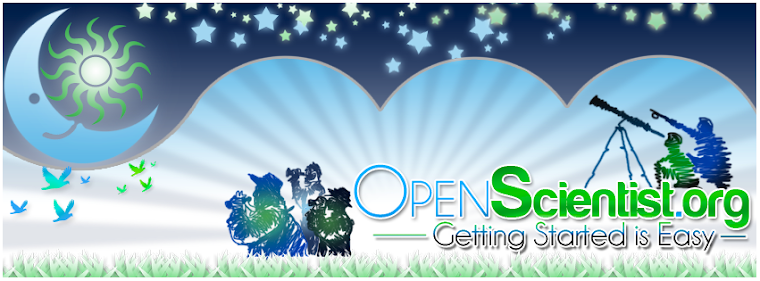
Tuesday, April 30, 2013
Research with a Humble Confidence - A Key to Citizen Science Ethics
Two short weeks ago the nation was shocked by the Boston Marathon bombing, and immediately we wanted to help. Many of us looked to give blood. Others sent donations to help the surviving families. And some wanted to ensure justice.
We saw this happen as people began combing through public footage to find clues the authorities may have missed. They set up Internet chat rooms and decided (on their own) to tap the public's collective wisdom and resources to find the perpetrators. In other words, they crowd-sourced the investigation. Users of the Reddit site quickly focused on one particular individual and began labeling him a potential suspect. Sadly, it appears they targeted an innocent man as we now know the Tsarnaev brothers were the guilty parties. But even worse is the fact that the notoriety quickly got to him to the point he was found dead last week. You can read much more about this episode on CNN (here), the Washington Post (here) or any other news web site.
At this point we still don't know what happened and his death may have been completely unrelated to the investigation. But it should make us all pause. There is huge promise and peril with opening research to the vast public. They can do great good or great harm. The key is do it all with a humble confidence.
One of my main goals with OpenScientist is boosting the confidence of the citizen science movement and encouraging everyday people that their contributions are meaningful. For too long society and academia have (inadvertently) sent this message and it remains one of citizen science's biggest obstacles to success. So I try to remember that every time I write a new blog post and I purposefully include different references to it. Sometimes it is showing all the famous scientists who began as citizen scientists. Sometimes it is showing the great discoveries made by average people. And other times it is showing that "Getting Started is easy" to help people start on their journey.
But the flip side to this confidence is humility.
We should not forget that while citizen science can match that of "professional" researchers, that doesn't mean to disparage the great work done in that realm too. Tenured professors may be the in an ivory tower, but they have also been studying their issues for years. They know the facts inside and out and have spent much of their life trying to develop new and innovative theories to move their fields forward. Their opinions should not be taken lightly. Citizen science should always augment their work and even question their work, but it must be done humbly and collaboratively. The beauty of science is that the facts speak for themselves. So we need to be part of the debate, not dominate it.
Crowdsourcing is a great part of that. This technique works fantastically well in the sciences as multiple eyes and minds can do higher quality work than a computer can, and at a faster pace. At that level having people scan video archives makes a lot of sense (and is similar to what many citizen science projects already do). Even taking that nest step of fresh analysis and hypothesis development is within the citizen science tradition. Though we are still figuring out how to identify good ideas from bad, there is still a lot of progress that can be made. But this has only been done with classifying galaxies or identifying plants...nobody is hurt if mistakes are made.
Handling error tolerance is an important design concept for crowdsourcing projects. This is where the Reddit users fell down. Once you start accusing a person of a horrific bombing it is tough to pull that back. Even if the public forgets, that person still carries it with them. So before we utilize a tool that can provide incorrect data, we need to have systems which correct that data or keep it in the proper context.
They also forgot the massive expertise brought in by the FBI and the Boston Police. Sure the investigators needed help...they often asked people to come forward with tips and for people with pictures/videos in that area to send them in. But they had crack professionals with years of experience to dissect, analyze, and properly follow up on all the leads.
In other words, we need to have confidence that users will find important data, but humble enough not to accuse people of mass murder without overwhelming evidence. And that we need to work with the authorities, not separate from them.
We see the need for humble confidence in the politics as well. The 24-hour news cycle and proliferation of government information have many great benefits. They keep everyone knowledgeable of world events, provide data to help them in their daily lives, and enable them to be fully active citizens in their democracy. With citizen science being dubbed by some the "Democratization of Science" the parallels are many. But like citizen science there are many downsides.
All of this information can also exacerbate our country's partisan divides and make our arguments even louder. Everyone has their own viewpoint and now their own data to confirm it. So people get louder and more self-righteous in their tone. After all, they have evidence to prove their point or disparage the other side. Why look for more?
Again, we need people to participate vigorously but with a humble confidence.
Policy is hard. Understanding what laws to enact to create a desired outcome is exceptionally difficult. It requires knowledge of sociology, economics, science, and human nature. And that's just for the easy ones. Add science-intensive topics such as global warming or public health, and the ability to know the "right answer" becomes mind-bogglingly hard.
So all I ask is that we call keep moving forward and do the amazing work we are capable of. Just remember that we are here as part of the discussion...we aren't the only discussion. We need to listen to other viewpoints and be cautious in making outrageous claims until the weight of the evidence forces us to. In the end the truth wins out. We just need to give it time.
Sunday, April 21, 2013
Wiki Surveys and the Suggestion Box Problem
I'm still looking at the Suggestion Box Problem and finding ways citizen science can capture the breadth and depth of ideas available from the public. There are so many ideas of varying quality and in vastly different areas it seems impossible to sort through them all. But I'm determined to find some models that can.
Beginning my research I stumbled upon a working paper published by Matthew J. Salganik and Karen E.C. Levy titled "Wiki Surveys: Open and Quantifiable Social Data Collection" (arXiv:1202.0500v1). In it they compare different methods social scientists use to collect data from the public, and then go one step further to describe a new web site (www.allourideas.org) to implement their ideas and prove the value of "Wiki Surveys". This seems like a great starting point.
I've highlighted a few key points from the article and offer some of my own thoughts on it's applicability to citizen science. But I also recommend reading the full working paper yourself, available for free here.
The authors begin by looking at the problems with different ways of collecting data.
While surveys allow researchers to quantify large amounts of information quickly and at a reasonable cost, they are routinely criticized for being "top-down" and rigid; that is, the survey questions and possible responses are formulated before data collection begins, meaning that surveys generally are not open to novel or unexpected information from respondents. In contrast, interviews allow new information to "bubble up" directly from respondents, but are slow, expensive, and difficult to quantify...Advances in computing technology now enable a hybrid approach that combines the quantifiability of a survey and the openness of an interview; we call this new class of data collection tools wiki surveys.
The authors also describe the trade-offs involved in designing a collection instrument:
The primary advantage of closed questions is that responses can be handled with relative ease: answers can be assigned values, fed into statistical software, and employed in quantitative analysis. There processes are relatively straightforward, fast, and inexpensive, making closed questions an efficient choice for large-scale social science surveys. In contrast, responses to open-ended questions are more complicated for researchers to reliably code and quantify...In some cases, however, open methods may provide insights that closed methods cannot because they are receptive to new information that was unanticipated by the researcher...Because respondents have a strong tendency to confine their responses to the answer choices offered (Krosnick, 1999; Schuman, 2008), researchers who construct all the possible answer choices necessarily constrain what can be learned. This is unfortunate because unanticipated information is often the most valuable for research.
Looking at our own field, citizen science seems to rely much more heavily on the first type of collection type, closed questions, and must less on open-ended questions. In fact many of the most popular citizen science programs involve public participation by collecting defined sets of data or answering specific sets of questions. We see this in tools such as eBird, Nature's Notebook, and the many other survey instruments that ask people to collect and report wildlife data that can be . There are also specific advantages to closed questions in citizen science, since often the public does not know what to look for or how to report it, since they are not highly trained in that field. In these cases the structure of closed questioning helps them organize their thoughts and narrow their focus. It also allows data to be transmitted electronically and stored as structured data items, helping analysis and accommodating mobile applications. So for many projects this can be a highly successful approach. But it still means many things may be missed.
Traditional surveys attempt to collect a fixed amount of information from each respondent; respondents who want to contribute less than one questionnaire's worth of information are considered problematic and respondents who want to contribute more are prohibited from doing so. This contrasts sharply with successful information aggregation projects on the Internet, which collect as much or as little information as each respondent is willing to provide. Such a structure typically results in highly unequal levels of contribution: when contributors are plotted in rank order, the distributions tend to show a small amount of heavy contributors -- the "fat head" -- and a large number of light contributors -- the "long tail" (Anderson, 2006; Wilkinson, 2008).
The Zooniverse projects have had success combining types of questions. Most of their projects break down large scientific tasks into smaller pieces and walking participants through a series of questions to describe a piece of data (picture, light curve, whale song, etc.) in a structured manner. But they also encourage people to identify "unknown" or "interesting" aspects of each picture they are reviewing, and they also have discussion forums that allow users to discuss interesting items not captured by the project tools. This is how they stumbled on Hanny's Voorwerp though there are also other examples. Interestingly, the paper authors discuss this type of approach in their background:
[Yochai] Benkler (The Wealth of Networks: How Social Production Transforms Markets and Freedom; 2006) notes that successful information aggregation systems are typically composed of granular, modular tasks. That is, in successful systems, large problems can be broken down into smaller "chunks" which require low individual investment of time and effort (granularity) and these "chunks" can be independently completed by many individuals before being flexibly integrated into a larger whole (modularity).
In developing their model the authors propose three traits that any successful survey instrument needs to have to capture information from a wide number of people and perspectives. Although these are applied to the Wiki Surveys they may also have wide use for our citizen science "Suggestion Box" problem and will be a useful tool in evaluating other potential approaches. The proposed key traits are:
- Adaptive: They should be continually optimized to elicit the most useful information for estimating the parameters of interest, given what is already known.
- Greedy: They should capture as much or as little information as a respondent is willing to provide.
- Collaborative: They should be open to new information contributed directly by respondents that may not have been anticipated by the researcher, as often happens during an interview. Crucially, unlike a traditional "other" box in a survey, this new information would then be presented to future respondents for evaluation. In this way, a wiki survey bears some resemblance to a focus group in which participants can respond to the contributions of others.
For example, let's say you want to find out what the most popular color is. As a researcher you seed the survey with Red, Orange, Yellow, Green, Blue, Indigo, and Violet. When a user logs on they are asked "Which of these two is your favorite color?" and they are forced to choose between Red and Indigo. Then Orange and Blue. Then Red and Orange. The questions continue and slowly the system can rank responses based on how people respond. Additionally, someone could add their own favorite, Maroon. The system will add now include Maroon as part of the randomly-generated pairs, so some users will be asked to choose between Indigo and Maroon, or Yellow and Maroon.
The AllOurIdeas site has actually hosted many surveys since the paper was first published, with over 2.5 million votes cast over 1,500 surveys. There were also over 60,000 new ideas submitted that the individual survey designers had not initially included and presumably never thought of. This includes two specific surveys, one for the New York City Mayor's Office on creating a greener, greater city and one for the international Organization for Economic Cooperation and Development. They noted a couple of interesting findings.
One is that "...wiki surveys are best suited for situations in which there is a single predetermined question." In some ways this fits the needs of citizen science projects since many currently exist with well-defined questions. But it doesn't hit our biggest problem...capturing chance discoveries and unexpected results. Some of the most powerful discoveries have been made accidentally while a scientist was looking at a different problem, or made a "mistake" in an experiment. Just think of the discovery of Penicillin or invention of the microwave...none of these was planned for but they became very important. It also doesn't capture theoretical discoveries or insights, just data. So the model does require continued development.
Additionally, it was discovered that:
...in both wiki surveys, many of the highest scoring ideas were uploaded by users. For PlaNYC, 8 of the top 10 ideas were uploaded by users, as were 7 of the top 10 for the OECD. ..There seem to be two general classes of uploaded ideas that score well: novel information -- that is, new ideas that were not anticipated by the wiki survey creators -- and alternative framings -- that is, new and "stickier" ways of expressing existing ideas.
All of this has given me some great ideas on how to use wiki surveys for my own research on citizen science topics. But I'm getting ahead of myself; there is still a lot more research to do first. Hopefully this has given you some insights too, and let me know if there are other papers you find that are also interesting. I'm still working through some more but would welcome any additional ideas.
Just let me know in the comments below.
Friday, April 12, 2013
Citizen Science and the Suggestion Box Problem
Citizen Science is about tapping the energy and knowledge of everyday people to advance our understanding of the world. This is great when we talk about organized projects that provide the public specific tasks or ask very specific questions. But how do we get to the next level? How do we answer the biggest questions and find the most creative solutions?
It all revolves around the Suggestion Box problem.
I've stolen this idea from the business world but I think the analogy works. All companies want to improve and keep their costs low. One way to do this is through plentiful input from workers. But "culling the wheat from the chaff" and identifying the few good ideas from the numerous bad ones is much tougher. A great example is in this Forbes article.
Imagine a company looking to reduce costs in their factory asks their workers for their best ideas. Each person takes a slip of paper and over the course of the day writes down every idea they have for saving money. After a week they all place their ideas in a suggestion box for management toreview. Now put yourself in the manager's place. Going through all those ideas many will be poorly conceived, expensive to implement, overly ambitious, or just plain bad ideas. You want to throw these out. But somewhere there is a fantastic idea so you wade through them all until the perfect one rises to the top. In a small firm with ten people that is easy to do. But make it 50, 100, or 10,000 people and it quickly becomes quite difficult.
Putting it back in science terms, we are all looking for the next Einstein....a brilliant patent clerk with a head brimming full of ideas ready to upset our entire understanding of the Universe. But even if this person exists, how will we find him? We can't just have him publish articles in physics journals to be noticed....he has no idea where to submit or have the ability to write an article that will pass peer review. He can't go to the press since they'll just dismiss him as a quack. And he can't necessarily create a new invention or create a huge demonstration...his insights are theoretical and aren't actual devices. How do we get that idea discovered? That's what I'd like to find out.
For starters I'm looking at research papers in a number of fields (including business, economics, sociology, politics, etc.) to see what insights already exist. I also know of some smaller-scale examples from the citizen science world that should help. But before I start writing about them...do you have any ideas? Tell me your best insights or show me some papers where you think some good ideas exist. Let's get those thoughts bubbling up and test the whole process in the comments below.
It all revolves around the Suggestion Box problem.
I've stolen this idea from the business world but I think the analogy works. All companies want to improve and keep their costs low. One way to do this is through plentiful input from workers. But "culling the wheat from the chaff" and identifying the few good ideas from the numerous bad ones is much tougher. A great example is in this Forbes article.
Imagine a company looking to reduce costs in their factory asks their workers for their best ideas. Each person takes a slip of paper and over the course of the day writes down every idea they have for saving money. After a week they all place their ideas in a suggestion box for management toreview. Now put yourself in the manager's place. Going through all those ideas many will be poorly conceived, expensive to implement, overly ambitious, or just plain bad ideas. You want to throw these out. But somewhere there is a fantastic idea so you wade through them all until the perfect one rises to the top. In a small firm with ten people that is easy to do. But make it 50, 100, or 10,000 people and it quickly becomes quite difficult.
Putting it back in science terms, we are all looking for the next Einstein....a brilliant patent clerk with a head brimming full of ideas ready to upset our entire understanding of the Universe. But even if this person exists, how will we find him? We can't just have him publish articles in physics journals to be noticed....he has no idea where to submit or have the ability to write an article that will pass peer review. He can't go to the press since they'll just dismiss him as a quack. And he can't necessarily create a new invention or create a huge demonstration...his insights are theoretical and aren't actual devices. How do we get that idea discovered? That's what I'd like to find out.
For starters I'm looking at research papers in a number of fields (including business, economics, sociology, politics, etc.) to see what insights already exist. I also know of some smaller-scale examples from the citizen science world that should help. But before I start writing about them...do you have any ideas? Tell me your best insights or show me some papers where you think some good ideas exist. Let's get those thoughts bubbling up and test the whole process in the comments below.
Subscribe to:
Posts (Atom)